Economic Simulations: A Canadian Proposal
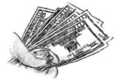
For some time now there has been concern over our beloved PowerPlay Manager's economic system specifically why people still aren't promoting when given the chance. In a recent article posted by the developers in the PPM Mag they stated that they will be undertaking addressing the current economic issue. In this article I wish to present one idea presented by the Canadian PPM community. While its idea in principle was not new to me, I must give credit to Elpatzer2 and Chinook for presenting and laying the foundation for this potential solution. I wish to emphasize that what I am going to write about here is by no means the best solution. It is merely one idea that I wish to share with all of you in hopes that more ideas will flow.
So what is the idea you may be asking? Well, it works off an ELO rating system. A system like this is used in chess and would be used to modify our current economic system. It has been clear that the developers do not wish to get rid of Overall Team Rating (OTR) as a means of conducting various calculations. However, under the current system, a weak team in a given league gets roughly the same amount of OTR (within 5%) for beating a strong team in its league as that strong team gets for beating it. The developers took a great first step by implementing different OTR gains based on league level, however we wish to take it a step further. The goal of this proposed system is to persuade teams to promote, specifically teams that are much stronger than the majority of its competition. Parallel to this, the weaker teams should get some form of advantage if they were to stay in the current league compared to relegating. This will give all teams an incentive to play in the best league possible and stay there.
Before I get into the details, I wish to discuss a few "disclaimers". The simulation program I wrote is a means of illustrating how a theory can be implemented. It in no way, shape, or form should be taken verbatim in any quantitative form. I have no way of knowing what the probability of Team A with OTS N will beat Team B with OTS M making it difficult to do an accurate simulation. However, based on this and a few other assumptions I was able to illustrate how an ELO system merged with OTR could be a viable solution. Furthermore, there are some simplifications that were made that need to be mentioned.
First, the simulation was run for a static OTS. That is, the team strength of each team did not grow throughout the entire process. This will skew the results in a sense that the amount of OTR gained for a win is based on the OTS ratio of the teams at the time of gameplay. This ratio would grow in favour of the stronger team as they'll have the better facilities to improve faster. However, this would reduce the OTR gained when the stronger team beats the weaker team thus changing the outcome quantitatively but not qualitatively.
Second, there was no promotion or relegation considered. While this could be implemented it would not do much in terms of viable change. The strongest team would promote and the weakest team would relegate thereby creating an effective swap. By not including this, all that it negates is observing how a weak team moving up a league with more OTR would do. However, given the plots to be attached later, this can be extrapolated with ease.
Lastly, this simulation does not consider OTR gained from friendlies and cup games such as the National Cup and International Cups. This is important to mention. There will likely be concern that under a system like this, the top teams in the top leagues would get hurt. Losing lots of OTR especially in countries where there is a clear dominant team. However, given these teams are the ones that go far in the National Cup and get the opportunity in all likelihood to represent the country at the international level, the decrease in their OTR from one season to the next would not be as drastic as portrayed. In all actuality, they'd likely reach an equilibrium 10% above what is illustrated come season 5.
So, now that I have that preamble out of the way, let me explain in more detail and show you what I'm talking about exactly. The algorithm for the theory is simple, the amount of OTR a team gains is primarily a function of their current strength and secondly of what league level they are in. These equations can be better described given the actual PPM simulation engine is put in place. Outside of this, teams lose OTR at the end of each season as they do now. Now lets look at some league-specific results. Please note, the initial OTR was set as a linear scale increasing from lowest OTS to highest OTS at season 0.
Canada III.2
I had a look at Canada's III.2 division and took note of their immediate OTS, regardless of whether they had players in a training camp or not. The same was done with the following leagues as well. Setting up the initial OTR to vary from 150 to 350 and plugging in the OTS for the 20 teams (ranging from 39 to 116) the simulation was run and the outcome can be observed below. At the end of the first season, all teams OTR increases. This is expected as the system has not had a chance to settle just yet. However, over time one can see that while the bottom three OTS teams are up the settle around their initial point (they'd actually be up at this point given friendly games and other means of obtaining OTR). The top two teams on the other hand are way down and this is what was hoped given their OTS dominance over the league median (green line). Again, these two teams will be higher given OTR obtained via other avenues, but it is clear that the longer the excessively dominant teams stay in this league, the more OTR hit they will take. Let's have a look at a second division league now and how a 116 OTS team would fare there under this system.
Canada II.4
Much like III.2's simulation, II.4 observed a similar trend. The strong teams took the most hit while the weaker teams improved their OTR. Now let's take the top team from III.2, at 116 OTS and around 375 OTR at the end of season 1. Considering the OTS of that team and the red team in the plot below are roughly equal, one can see that a promotion would be beneficial (map this promotion team to just below the blue line and compare to the OTR it would have had it stayed in III.2 at that season). Again, it is hard to picture what will exactly happen due to the assumptions and simplifications but the underlying premise is what I want to illustrate. In hindsight I shouldn't have placed OTR labels and simply used an arbitrary scale as they are meaningless given the current assumptions and simplifications. I do hope that you are soaking this in and are understanding what I'm trying to explain.
Canada I.1
The setup I calibrated for I.1 I believe turned out the best. The top teams cannot promote anywhere, in turn they beat up on the same teams time and time again increasing their gap and dominance. Observing the plot below it is clear that this system closes the gap between the weakest I.1 teams and the strongest I.1 teams. This is especially a perfect example for second division teams promoting. Given an OTS from 130-140 (green line roughly), if the team could save off relegation they'd settle in the 450+ OTR region (higher yet given other means of gaining OTR). However, if they stayed in the second division, their OTS dominance would only grow even more hereby decreasing the OTR gained per win and plummeting their OTR well below the 350 region. This is what I feel best portrays the desired outcome.
In real life, a weak team in the top division is benefited more than a strong team in the second division. Under a system similar to this, this would be the case as the top teams would want to battle for not only promotion but also to stay in the highest league possible. I wish to emphasize again that this is merely a theoretical and qualitative simulation. Please be mindful of the assumptions and simplifications that made it possible to complete this simulation in a small time window. I know it'll likely get met with mixed reviews. I can assure you, given enough data and a proper simulation engine to base the governing model on, this system will work. Now, it may or may not be how the developers choose to address the current issue regarding economics. However, whatever the method it'll be well planned.
I hope you all have enjoyed my little story here. I'll be happy to address any and all concerns. It is hoped that this will get the creative juices flowing and get the voices of the community heard. Thank you and I hope you enjoyed your (long) weekend,
-Scott
Note: I have discovered a bug in my program post-publish. This bug is responsible for the spike observed in S1. Correcting this the OTR gradient from one season to the next is more fluid.
Sdílej na Facebooku Sdílej na Twitteri Sdílej na MySpace